Eye-tracking signals can be leveraged to provide an inductive bias to the computational language models, which can improve their performance. Additionally, they can be used to evaluate the inner workings of deep neural language models to increase their interpretability and explainability. For instance, the human attention patterns in eye-tracking data can be compared to machine attention mechanisms to gain a better understanding of the similarities and differences between human and computational language processing. WG4 will investigate the potential applications of eye-tracking data in natural language processing. The WG will study the cognitive plausibility of multilingual language models by providing a method for intrinsic evaluation as well as opportunities to analyse the similarities and differences in human and machine attention. The WG will further study how eye-tracking data can be used to improve transfer learning between similar languages as well as from a high-resource to a low-resource language. The large eye tracking data collection will enable researchers to study machine translation acceptability, and to develop personalized text summarization. WG4 will study to what extent eye tracking data can be used as human rationales to evaluate the decision-making process of NLP systems and to provide ground truth labels for machine learning models. More human-centered NLP applications will improve the usability and acceptability of modern language technologies.
Deliverables
- Publications in NLP conference proceedings and journals including a review paper summarizing the applications of eye-tracking data in NLP
- Training School and Workshop in machine learning and NLP for linguists
Leader
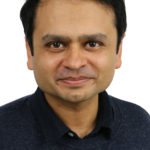